インタラクティブメディア設計学研究室助教の澤邊太志です。本研究室では、コンピュタで作られた情報を実世界に重ねあわせて表示する拡張現実間(AR)技術を中心としつつ、VR(バーチャルリアリティ)、CV(コンピュータビジョン)、CG(コンピュターグラフィクス)、HCI(ヒューマンコンピュータインタラクション)、HRI(ヒューマンロボットインタラクション)について幅広く研究しています。詳しく知りたい方は、本研究室HP(https://imdl.naist.jp/ja/prospective/)をご覧ください。今回は、私が関わっているHRIのロボットと人のインタラクションに関する研究を、3つご紹介します。
1. 快適自動走行:XRモビリティープラットフォーム
一つ目の研究は、快適な自動走行車(自律移動ロボット)を実現することを目的とした、自動走行車と人のインタラクション研究です。自動走行化することによって、従来運転手だった人も、搭乗者の一人となります。自動走行レベル5では、運転手を必要とせず、走行エリアも限定されずにどんな場所の道路でも自動運転で走行が可能な状態となり、より自由な空間が生まれると考えられています。しかしその一方で、自動走行車と私たち利用者の意思疎通が難しくなり、その結果、恐怖心や不安感などの精神的なストレス増加や乗り物酔い増加につながります。そこで私たちは、快適化知能(コンフォート・インテリジェンス)という、安全性や効率性だけでなく、人の快適性をも考慮した、新しい知能を作る研究をしています。その研究では、精神的要因であるストレスや生理的要因である酔いを対象に、その不快要因の推定や解析、軽減手法などの提案を行い、より快適な自動走行車の実現を目指しています。
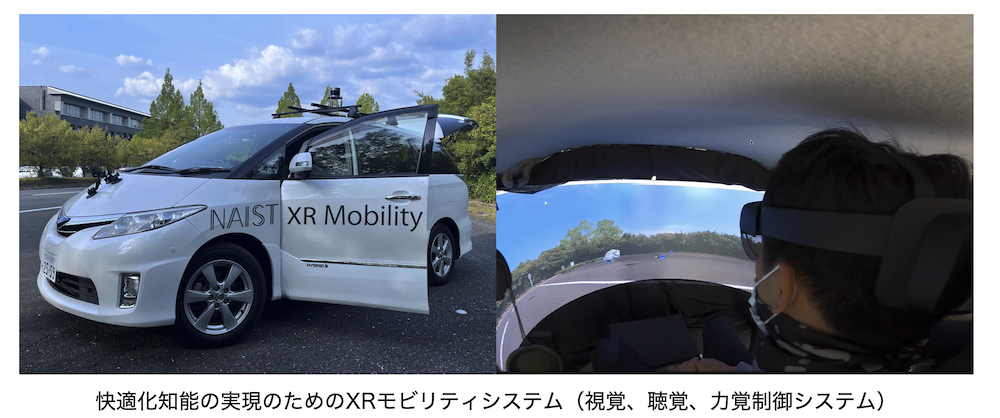
2. 快適なコミュニケーションパートナーロボット
二つ目の研究は、快適なコミュニケーションパートナーロボットを実現することを目的とした、ロボット(物理的ロボットやVR/ARアバタ)と人のインタラクション研究です。最近では、一人暮らしの若者や独居高齢者が増加していること、またコロナ禍ということより、以前よりも人と接することが難しくなってきています。そんな中、人との遠隔コミュニケーションや見守りという観点から、パートナーのような存在であるロボットのニーズが高まっています。パートナーになるためには、ロボットと人の信頼感が重要となってきますが、メカメカしい見た目のロボットや、カメラやセンサがいっぱい付いたロボットとのコミュニケーションは、やはり楽しさや面白さに欠け、継続的に利用するというのが難しくなります。そこで、私たちは、すでに生活の一部となっているような媒体(例えば、TVやゲームなど)を利用した対話ロボットによるインタラクション研究や、信頼感構築や継続意欲向上のための人の心理学的な知見(例、オペラント条件づけ)をもとにしたARアバタのインタラクション研究などを通して、信頼感を構築できるロボットインタラクションの研究を行なっています。
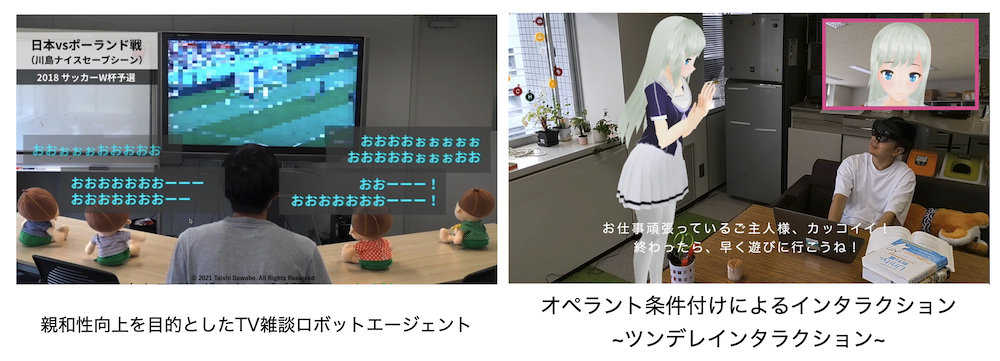
3. 快適なマルチモーダルタッチケアロボット
三つ目の研究は、快適なタッチケアロボットを実現することを目的とした、ロボットと人のインタラクション研究です。触れるということは、とても重要なことで、心を落ち着かせることができ(タッチケア)、人を幸せな気持ちにさせることができると医学的に分かっています。しかし、コロナ禍の影響で人と物理的に接することがより難しくなってきている現在、遠隔からでも人に触れて、安心感や幸福感を与えることができるケアロボットのニーズが高まっています。私たちは、快適なタッチケアのインタラクション研究を通して、触覚のインタラクショだけでなく、視覚や聴覚を含む五感に対して、マルチモーダルなインタラクションを行うことで、人の快適性を向上させるタッチケアロボットの研究を行なっています。
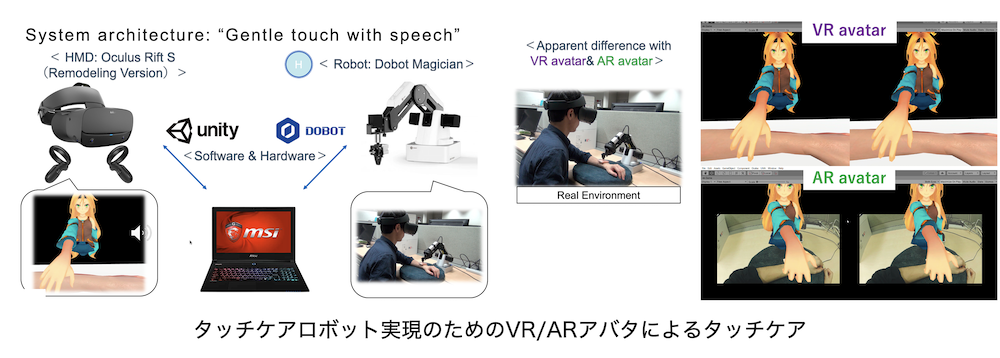
上記以外のテーマでも、様々な視点からロボットと人の快適なインタラクション研究を行なっています。少しでも興味がある方は、一度サイトをご覧ください。是非一緒に快適なロボットに囲まれた世界を作りましょう。
著者紹介
澤邊 太志(さわべ たいし)
大阪生まれ、オーストラリア育ち、立命館大学のロボティクス学科を卒業後、奈良先端科学技術大学院大学(NAIST)にて博士前期・後期課程を修了。博士(工学)。同大学ポスドクを経て、助教。博士課程時に大学発ベンチャーとして、㈱アミロボテック(https://www.amirobo.tech/)を大学の寮で設立し、京都のテック企業としても活動。HRIやVR分野にて、人とロボットの快適なインタラクション研究に従事し、研究基礎技術の応用化のためのアプリ開発等も行う。
🔗 Webサイト: https://drmax.mystrikingly.com/
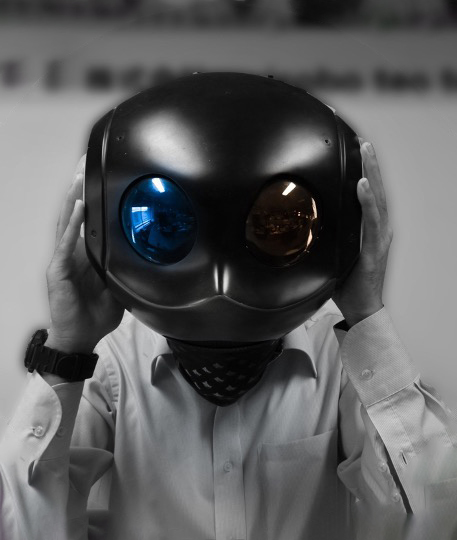